书接上文,我们使用 Python + Selenium 白嫖了原来要收费的图档清理大师,效果极好。然而,我们的代码还有很多可以优化之处,这就是写本文的由来。
方法优化:避免开启浏览器
在写完代码发完朋友圈之后我才开始认真思考一个问题:
为啥不直接逆向在线体验的 API 啊?
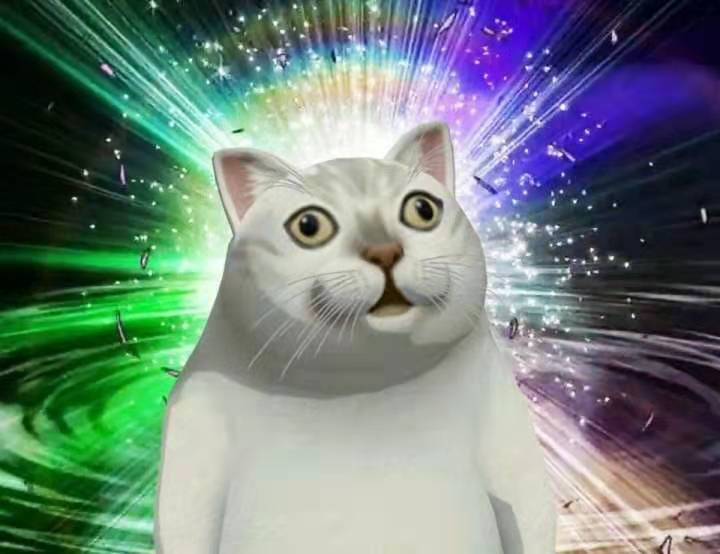
我之前自己把自己给绕进去了。
Selenium 模拟的好处是实现思路自然,只需要知道人工使用的方法而不需要知道内部的代码实现与网络通信。但缺点也很明显,需要开浏览器带来了一个明显的开销。浏览器的内存占用使得并行操作也存在一定的难度。
而直接模拟网络请求的好处就是确保没有资源浪费,存在并行化的潜质,而且相较于浏览器涉及到的不见耦合较少具有更高的稳定性(前提是 API 不变。Selenium 则相较之下在内部 API 变化但界面不变的情况下具备跨版本的稳定性)。
试一试?
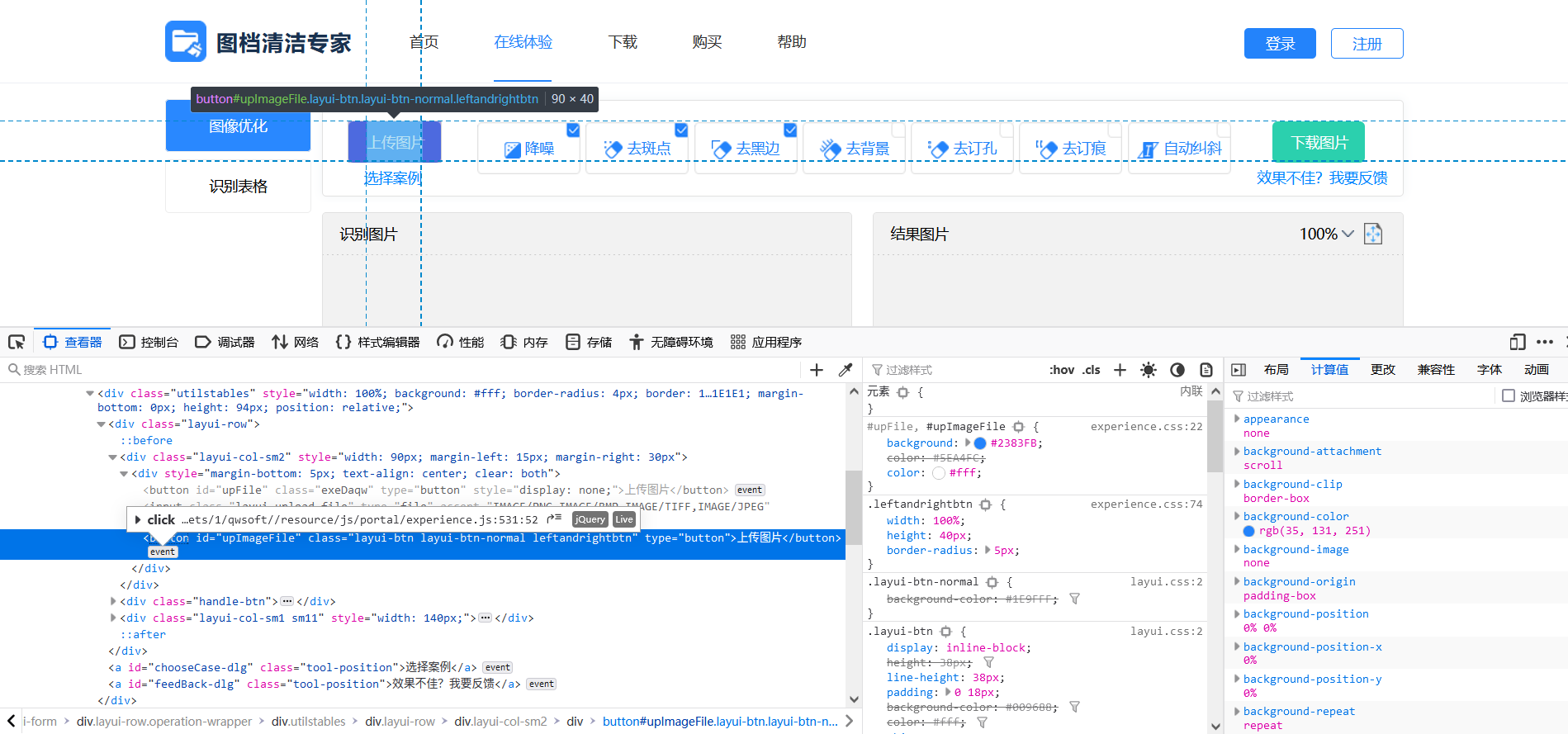
打开开发者界面,定位到上传图片的按钮,Firefox 的调试器很贴心地告诉我这里有一个 event,于是点进去。
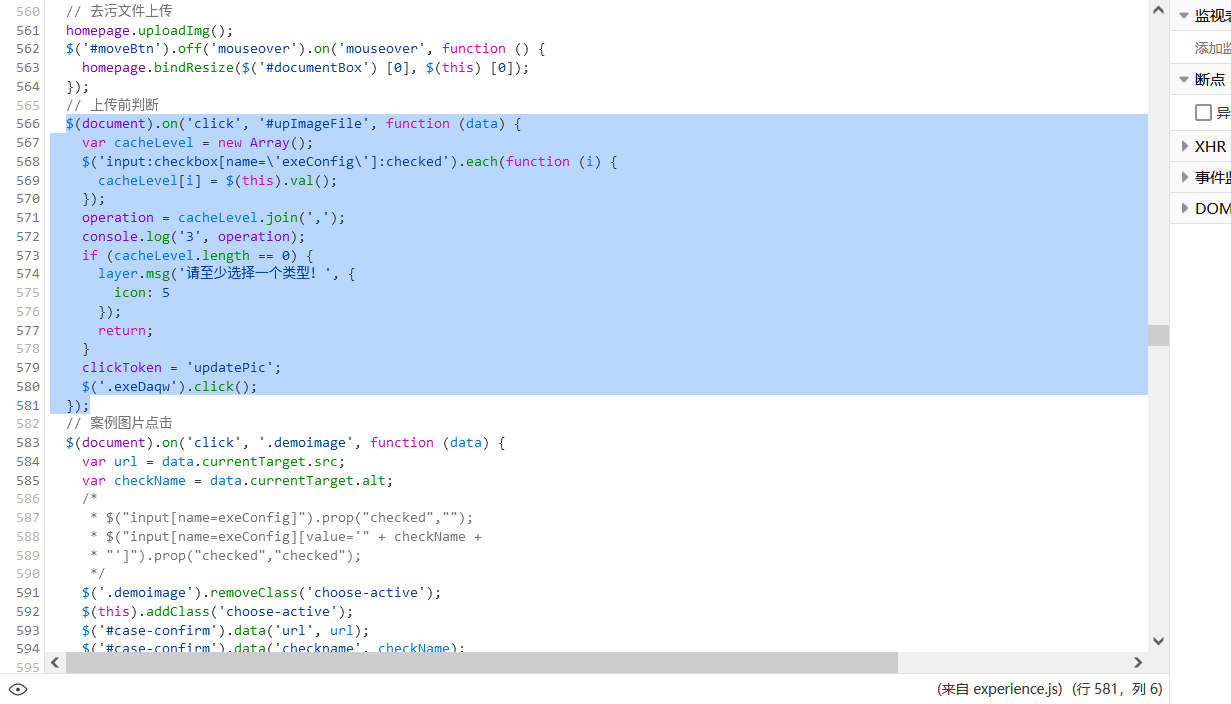
网站的开发者很贴心地没有做 minify 也没有做混淆,甚至还留了注释。
我发现上传的主要逻辑其实在 $('.exeDaqw').click()
里,于是进一步在源码当中搜索.exeDaqw
,发现如下片段:
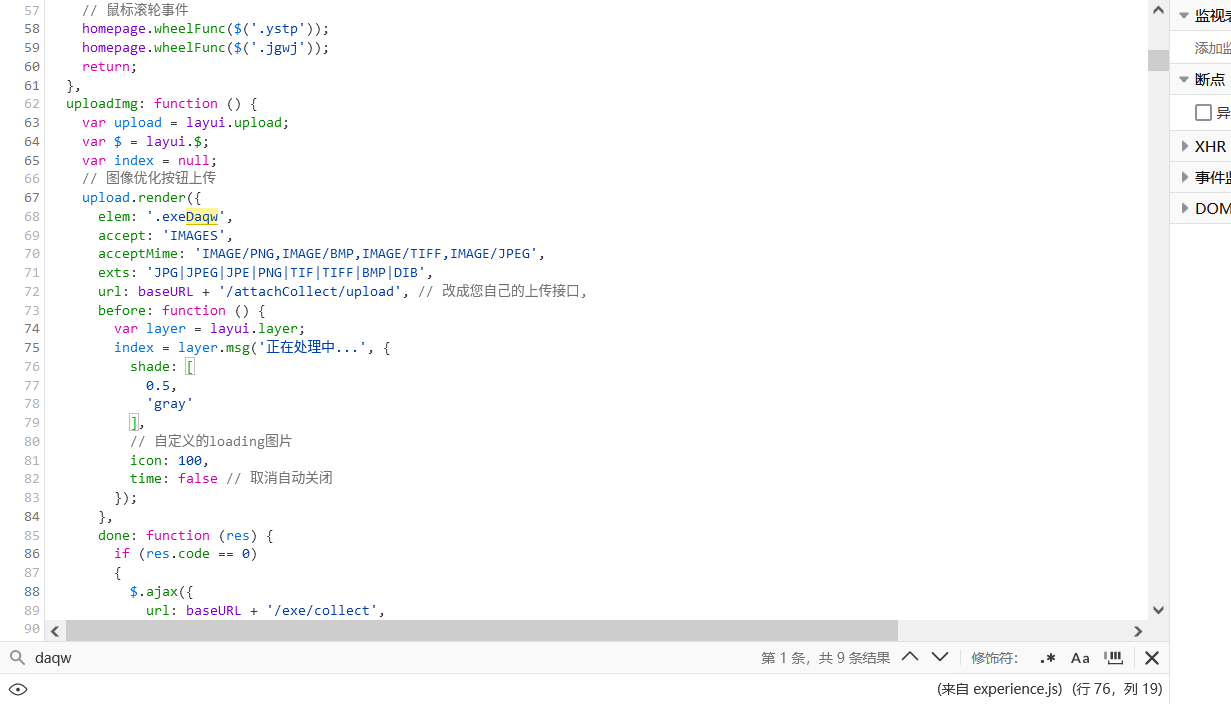
通过阅读片段可以发现,这个.exeDaqw
元素是通过 layui.upload
渲染的。我孤陋寡闻,但 Layui 看起来是一个控件库,事实也是如此。通过 url
字段我得以确定上传图片的 API 地址。在随后的代码当中又可以发现图片清理的 API 地址。
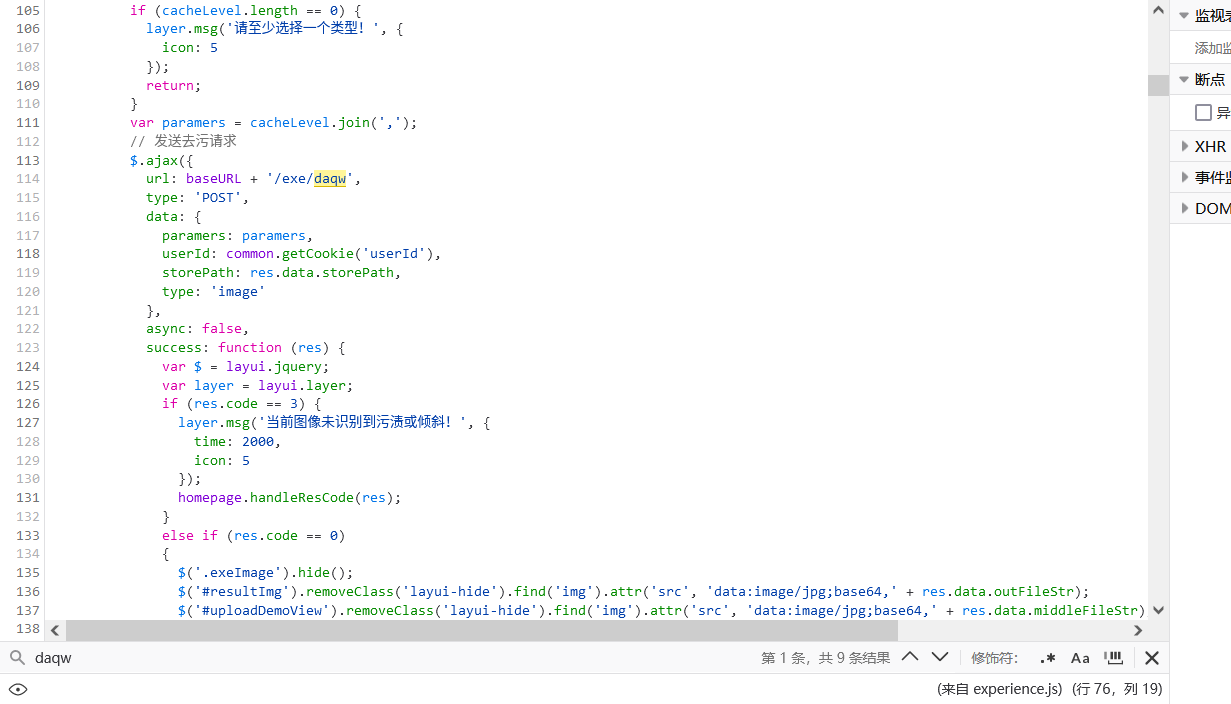
通过开发者窗口的网络监视器可以看到请求的实际情况:
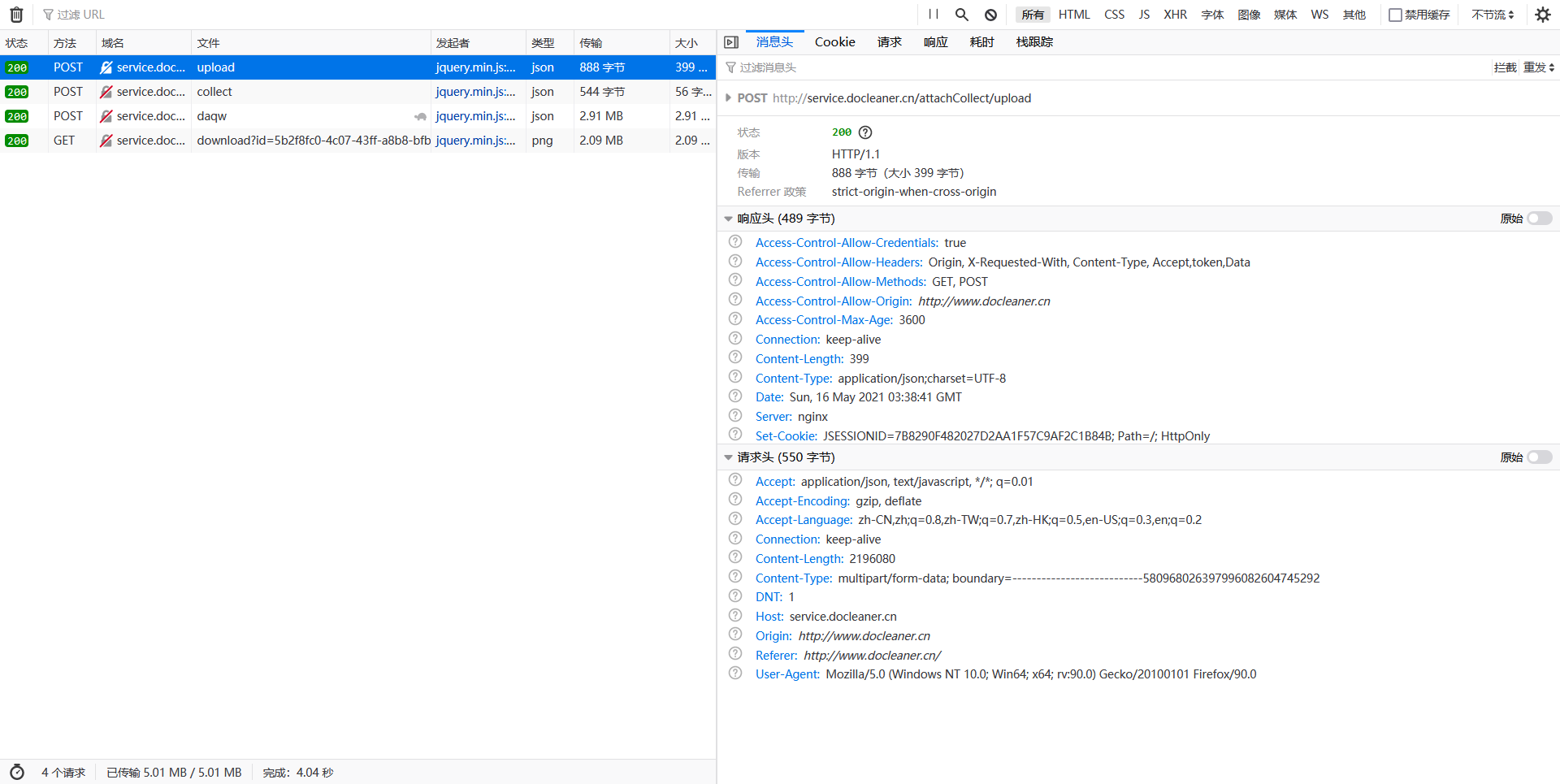
经过一波操作比对,摸索得到的 API 可以写成如下代码:
import requests
def clean_doc_requests(images: Generator[Tuple[bytes, str], None, None]) \
-> Generator[Image.Image, None, None]:
"""
Cleans the scanned document pages using docleaner's online service.
:param images: A generator yielding (an image as raw bytes, its extension as string).
"""
for (image, ext) in images:
# noinspection HttpUrlsUsage
= requests.post("http://service.docleaner.cn/attachCollect/upload",
req ={"file": (f"image.{ext}", image)})
files= {
data # Weird typo in the API.
"paramers": "降噪,去斑点,去黑边,去背景,自动纠斜",
"type": "image",
"storePath": req.json()["data"]["storePath"],
"userId": ""
}# noinspection HttpUrlsUsage
= requests.post("http://service.docleaner.cn/exe/daqw", data=data)
req = base64.b64decode(req.json()["data"]["outFileStr"])
result yield Image.open(io.BytesIO(result))
和之前上面的 clean_doc_online
代码对比,可以看到代码和逻辑确实都简洁了很多,这就是扒 API 直接用的优点了。我很幸运,这个站点的代码和注释都很清楚,所以逆向还原出 API 还是很容易的,对于更加复杂的一些站点,Selenium 可能不失为一个更简单粗暴的好办法。
但有意思的地方来了:上面的这串代码,跑的比 Selenium 要慢。原因在之前解释 Selenium 的时候大致讲过了。Selenium 新开了一个浏览器进程,那里上传图片还是等待结果本质上不会阻塞 Python 的运行,所以通过优化循环的写法,可以上传图片和从 PDF 中获取下一页图片同时进行。而 requests
是 Python 库,在请求时是阻塞的,因此就慢了。
失之东隅收之桑榆,虽然我们的 “优化” 让我们的代码反而变慢了,但是也为进一步的优化铺平了道路:在用 Selenium 的时候,因为同时开几十个浏览器不仅视觉上很离谱而且内存占用很高,所以并行处理很难;而同时开几十个并发的请求却是再容易不过的事情了。因此我们下一步的优化就是利用 Python 的多进程库进行加速。
速度优化:多进程加速
Python 写并行的格局,是和别处不同的。究其原因,GIL 的存在硬是让多线程的并行成了并发。而绕过 GIL 的唯一途径就是多进程,这又涉及到了进程之间通信,同步等一连串复杂的逻辑,令人望而生畏(至少我是这样的)。
但 Python 有一个好 ——battery
included。我去官方文档转了一圈,发现 Python 的标准库里有一个 multiprocessing 模块,应付我这里的需求已经完全够用。具体来说,multiprocessing 提供了一个进程池 Pool
:对于一般的
= map(function, input_iterator) output_iterator
只需要改写成
from multiprocessing import Pool
with Pool(process_count) as p:
= p.imap(function, input_iterator) output_iterator
就可以把 map 的函数分派到线程池的多个线程上进行运算,并在结果出来之后进行保序归并,最终得到和 map
一样的结果。
为了让我们的代码可以套用这个模式,我们需要把之前生成器套生成器的逻辑重构成单个函数:
def clean_single_page(args: Tuple[StrPath, int, int, bool, bool]) \
-> Union[Image.Image, bytes]:
"""
Cleans a single page.
:param args: A tuple consisting of (in order):
1. Path to the page (pdf or image),
2. Index (image index or page index in PDF),
3. DPI (-1 if an image is direcly supplied),
4. Whether to perform OCR,
5. Whether to actually clean the page.
:return: If OCR is enabled, an OCR-ed PDF in raw bytes, otherwise a PIL
Image object representing the cleaned page.
"""
= args
page, idx, dpi, ocr, clean ...
如上所示,从 PDF 中提取页面图像、上传图像到图档清理大师进行清理、对于结果的 OCR 都是可以单独进行的,故合并。
逻辑的合并自然导致参数的合并,而 map
接受的函数应当是单入单出的,于是我们就需要把所有参数打包成一个 tuple 进行用,并在函数体内部解包,即上面代码的第 15 行。
把所有页面重新归并成 PDF 的函数也要进行一定的简化与修改:
def merge_to_pdf(pages: Iterable[Union[Image.Image, bytes]], output: StrPath):
"""
Converts and merges images to a one-page pdf file, performing optional
OCR in the process.
:param pages: A generator yielding PIL image objects.
:param output: Path to the result pdf.
"""
= fitz.Document()
doc for page in pages:
if isinstance(page, Image.Image):
# noinspection PyUnresolvedReferences
= doc.new_page(width=page.width, height=page.height)
doc_page buffer = io.BytesIO()
buffer, format="jpeg")
page.save(0, 0, page.width, page.height),
doc_page.insert_image(fitz.Rect(=buffer)
streamelse:
= fitz.Document(stream=page, filetype="pdf")
page
doc.insert_pdf(page) doc.save(output)
最后,从之前的代码应该可以看出,我在编写代码的时候一直把逻辑分得比较开,目的是在可能的情况下使脚本的使用能够更加灵活。例如,能不能以通配符的形式直接读取图片进行优化?能不能再输出的时候直接输出到文件夹中而跳过 PDF 归并从而便于其他软件?多进程的优化是一个重要的重构,因此趁这个重构的机会我也把之前提到的功能理了理和调用 Pool
的代码一起加在了 CLI 入口点:
@click.command()
@click.argument("input", type=click.Path())
@click.argument("output", type=click.Path())
@click.option("-d", "--dpi", default=300, help="DPI for rasterization.")
@click.option("--first-page", type=int, help="First page to convert/clean.")
@click.option("--last-page", type=int, help="Last page to convert/clean.")
@click.option("--ocr/--no-ocr", default=True,
help="Whether to perform OCR during the conversion.")
@click.option("--clean/--dont-clean", default=True,
help="Whether to clean pdf using docleaner's online service.")
def main(input: str, output: str, dpi: int,
int], last_page: Optional[int], ocr: bool,
first_page: Optional[bool):
clean: if os.path.splitext(input)[1].lower() == ".pdf":
# PDF mode
assert os.path.exists(input)
= fitz.Document(input).page_count
page_count = 0 if first_page is None else first_page - 1
first_page = page_count if last_page is None else last_page
last_page = zip(repeat(input), range(first_page, last_page),
args
repeat(dpi), repeat(ocr), repeat(clean))else:
# Glob mode
= sorted(glob.glob(input, recursive=True))
files = 0 if first_page is None else first_page - 1
first_page = len(files) if last_page is None else last_page
last_page = zip(files[first_page:last_page], repeat(0), repeat(-1),
args
repeat(ocr), repeat(clean))= last_page - first_page
total with Pool() as p:
= tqdm(p.imap(clean_single_page, args), total=total)
results if os.path.splitext(output)[1].lower() == ".pdf":
merge_to_pdf(results, output)elif not os.path.exists(output) or os.path.isdir(output):
if ocr:
raise RuntimeError("the OCR flag is useless because we are "
"writing images (not PDF) to the output "
"directory.")
if not os.path.exists(output):
=True)
Path(output).mkdir(parentsfor (index, page) in enumerate(results):
= os.path.join(output, f"{index}.jpg")
file_path assert isinstance(page, Image.Image)
page.save(file_path)else:
raise RuntimeError("invalid output format.")
在多进程优化之后,脚本花了 11 分 21 秒就把测试用的《态度改变与社会影响》一书全文清理完毕。和原来 30-40 分钟的耗时对比,显然我们的优化是卓有成效的。
大小优化
未完待续
现在唯一一个比较明显的问题就是:清理之后的结果文件实在是太大了。
究其原因,现在的脚本是将 PDF 按一定的分辨率光栅化之后获得图像进行清理,而不是直接从 PDF 中提取本来存在的图像。现在方法的好处是实现简洁,无需考虑 PDF 内图像的存储方式(测试中发现直接提取 PDF 中图像提取出来可能是横的,这就说明 PDF 其实再显示的时候额外标注了旋转的信息,这在直接提取中会丢失),但坏处就是结果图像的大小和原本嵌入的图像大小无关,而且提取的过程中可能产生误差 (artifact,不知道怎么翻最为恰当)。
因此,解决这个问题也有两种思路:
- 治本:使用更复杂一点的逻辑对 PDF 中内嵌的图片进行保真的提取。这样一来结果文件的大小就和原来的文件大致一致,可能还会更小一点(考虑到清理的时候很多区域都会整个变成白色)。
- 治标:使用更加优秀的图像压缩算法。
第一种思路还未实验。关于第二种思路,我不禁想到 Google 出品的无脑图像压缩应用 Squoosh。Squoosh 提供 CLI,而且压缩率可以达到 30%-40%,很有的吸引力。进一步探究,Squoosh 默认使用的压缩工具是 MozJPEG。这款 Mozilla 出品的压缩编码器似乎也是唯一靠谱的我们脚本里能用的(Squoosh 里其他的压缩器的输出格式无法嵌入到 PDF 中,而且耗时太长)。但我在 Windows 上测试 MozJPEG 的 CLI,发现无法输出合法的 JPEG 文件。还在尝试中。